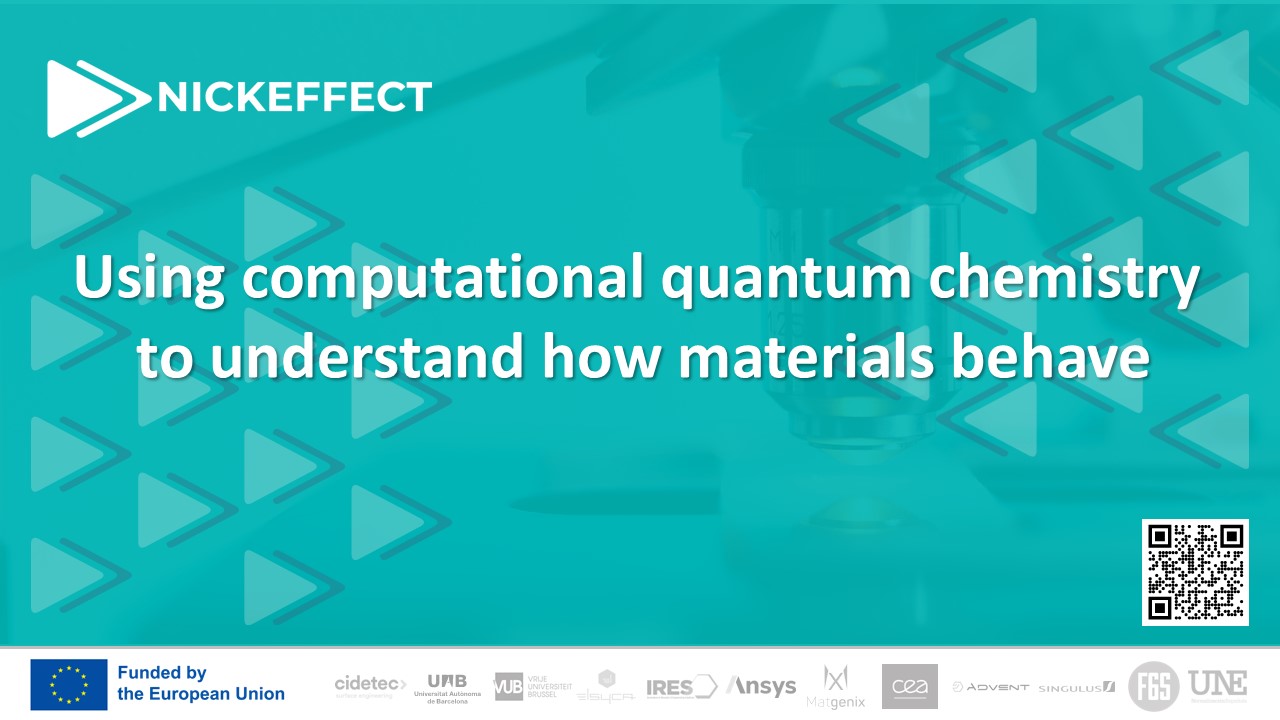
Materials selection is at the heart of the NICKEFFECT project. Replacing Pt-group metals with Ni is far from trivial. Materials with new compositions, structures, and topologies have to be explored and their physical and chemical properties need to be assessed. Traditionally, this exploration has been performed experimentally: a material is first synthesized and then tested in a lab to check whether it fulfills the requirements related to its application. This approach is long, requires resources, and can lead to failure at any step of the process. The scientist iterates through materials until a good solution is found, through trial and error or serendipity.
Fortunately, in the last few decades, computational tools have reached a maturity where the stability and physical properties of materials can be predicted before synthesizing them. These tools rely on density-functional theory (DFT) or more recently on machine learning (ML) when data is available. Such computations are not trivial and typically require a good amount of expertise. However, in the last few years, tools have emerged to automate the steps of such computations so that many chemistries can be tested in silico in a high-throughput fashion. The computational quantum chemist or solid-state physicist can then browse the materials and select the few most relevant ones for the next steps.
Once a few materials have been selected from a vast pool of possible candidates, multiple routes can be taken to go further. Either these candidates are directly tested experimentally (i.e., experimentalists try and synthesize them and then test whether the predicted properties are confirmed or not), or additional computations can be performed. Indeed, the automated computations using DFT typically describe the ground state of the materials. These are relatively cheap computations in terms of computing power. More advanced properties can however be predicted, such as reaction pathways, energy barriers for ion motion within a crystal, defects characterization, excited-state properties, heat and electronic transport, or optical properties. Higher-accuracy methods can also be used to better quantify materials properties. These computations are typically more computationally demanding, and most have not yet been automated in existing frameworks.
In addition to guiding experimentalists towards the best compounds, such first-principles computations can be used for existing materials to better understand their properties or the physical phenomena at play that are observed in the lab. At Matgenix, we use our expertise in DFT and ML computations and characterization of materials to help other members of the NICKEFFECT project understand what happens when Pt-group metals are replaced by Ni in known compounds. For this purpose, we use state-of-the-art, high-accuracy methods that require large computational resources. The computing power is mainly supplied by Lucia, the recent Walloon supercomputer (356th in the top500 list), and Lumi, the biggest supercomputer in Europe (5th in the top500 list).