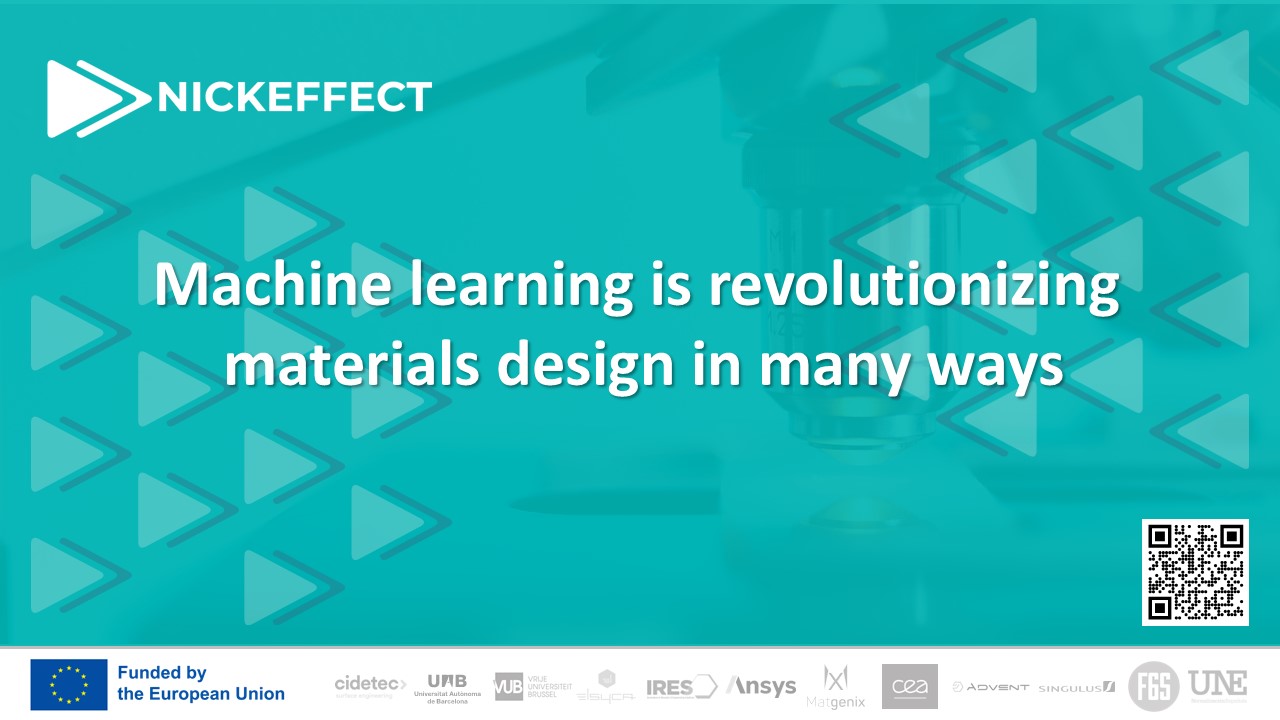
Modeling materials from first principles, i.e., without the need to fit or depend on experimental data, has taken on great importance in the last twenty years. Such methodology allows a deeper understanding of the physico-chemical phenomena dictating how materials behave. Density-functional theory (DFT) has been the tool of choice for computing the properties of materials at the nanoscale for decades. Indeed, ground-state properties such as phase diagrams, magnetism, or band gaps (determining whether a material is an insulator, semiconductor, or metal) and structures as well as more advanced properties such as charge carrier mobilities, ionic conductivity, or light emission/absorption spectra are all reachable within DFT and its extensions.
Because such computations are very demanding, both in terms of CPU power and human efforts, in the last 15 years additional tools have been developed to handle hundreds of thousands of such calculations and store them efficiently in databases. For example, the Materials Project gathers computed properties for thousands of materials. Using those databases, it is possible to scan and filter hundreds of thousands of materials, looking for the ones satisfying a series of requirements for a given application (e.g., the material should be thermodynamically stable, the band gap should be greater than 3 eV, it should not include toxic or rare elements, etc.). The material candidates passing through the filters can be further investigated, either theoretically (with more advanced computations) or experimentally. This approach has shown great success for materials design in various technological domains.
In the last few years, machine learning (ML) has become a great tool for the material science community. It has already been successfully used for, e.g., optimizing material deposition processes to reach enhanced material properties, as proven in the framework of the NICKEFFECT project. Machine learning regression models have also been used to predict the properties of new materials based on their composition and/or crystal structure. The training dataset for such regression models can be experimental or theoretical (e.g., the Materials Project), and some methodologies even allow to combine multiple datasets with different levels of fidelity (i.e. how close it is to the real material). A third approach has been to use ML to model the interactions between ions in crystals. A so-called machine-learned interatomic potential (MLIP) describes the forces exerted on each atom depending on their environment (i.e., the locations and nature of other atoms around them). The knowledge of the interatomic potential in a material allows to describe dynamical properties such as ionic diffusivity. It also makes it possible to find new stable materials that have not yet been explored, unveiling entire new pans of the chemical space. The advantage of MLIPs over first principles interatomic potentials is speed: once trained, a MLIP can predict the forces and dynamics of a system thousands of times faster than DFT does. Larger systems can therefore be studied than what is possible with DFT. The advantage over classical interatomic potentials is that MLIPs keep the precision of DFT, far greater than what classical potentials are able to perform. Additionally, MLIPs do not require fitting the potential using experimental data, only DFT computations are needed.
Those advantages of MLIPs compared to classical or DFT interatomic potentials, together with the advent of databases containing millions of DFT computations, has led to the training of so-called universal MLIPs, also called foundation models. These are potentials that have been trained on data including most of the atoms of the periodic table. This adds another great advantage: transferability (or universality, as the name suggests). The same potential can be used for any system, including any type of atoms, at a fraction of the cost of DFT computations. Many universal MLIPs have appeared over the last 4 years, with recent efforts even from Microsoft (MatterSim) and META (OMat24). At Matgenix, we use these foundation models, compare their performances, and enhance their results through fine-tuning. They help us describe the dynamics of complex systems in an efficient way, as shown in the case of Ni and Co oxides in the framework of NICKEFFECT.